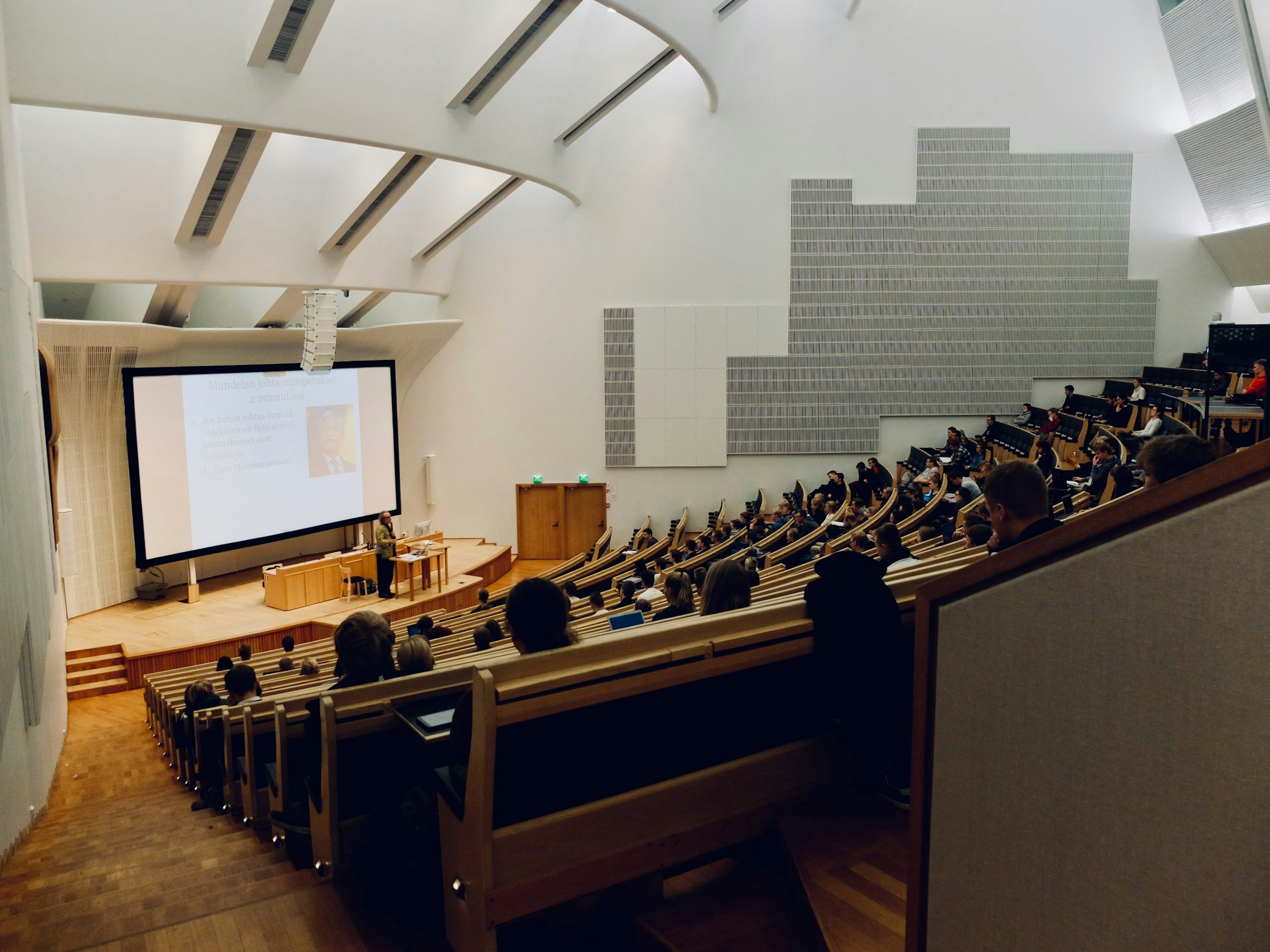
Academic Research
Our team are experts at building science, machine learning, and artificial intelligence. Our Research & Development team shares our research in academic journals.
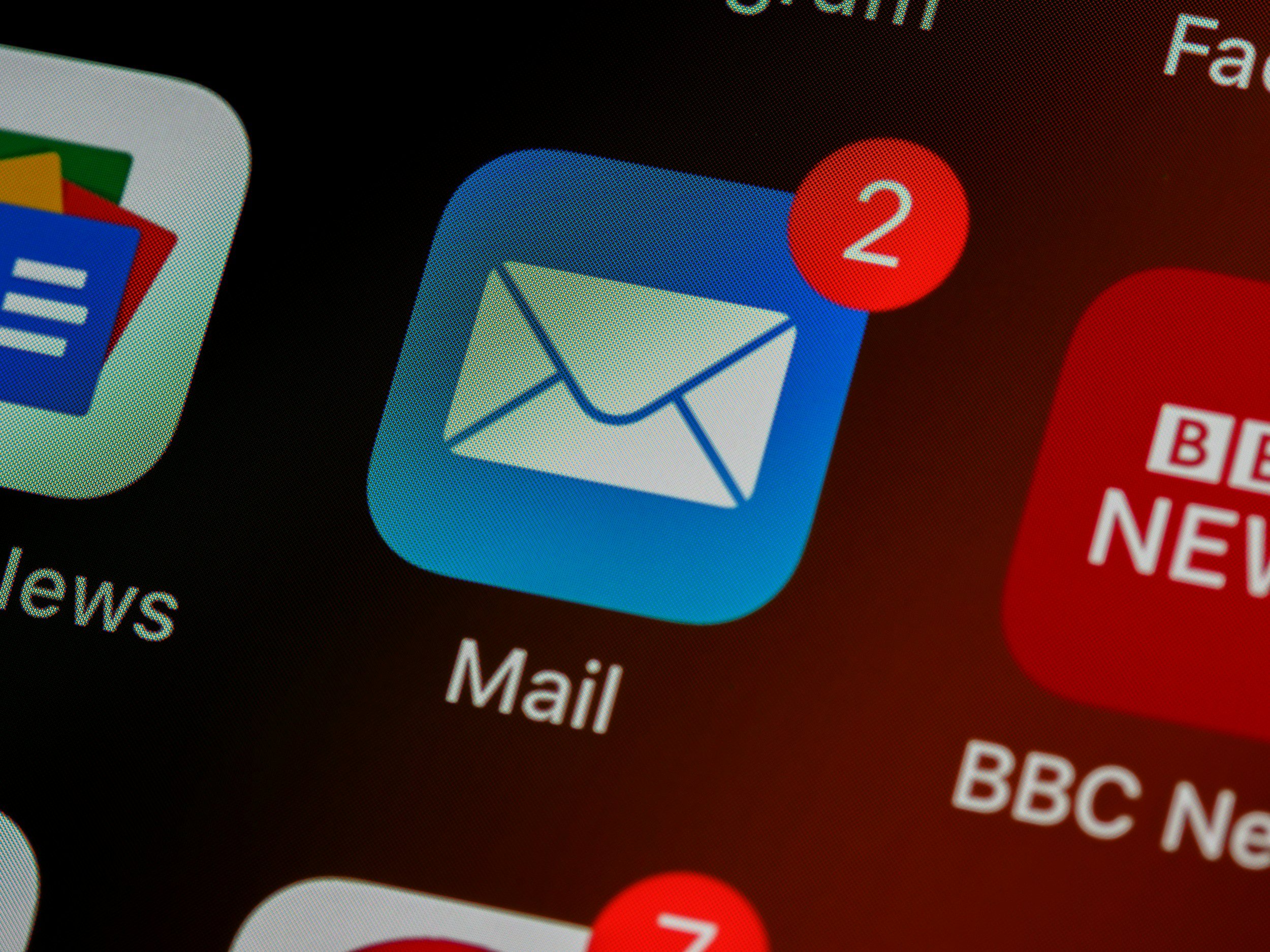
Newsletter Subscription
Sign up to stay informed with the latest updates, exclusive news, future events & opportunities, and valuable insights delivered directly to your inbox.
Demand-Side Management Under Real-Time Greenhouse Gas Emission Factor for Electricity
Electric power generation contributes to the second largest share of greenhouse gas (GHG) emissions in the US. The direct and indirect carbon emissions created from generating electricity vary from resources of generation, and power plant efficiency. Approximately 60% of the electricity comes from burning fossil fuels, mostly coal and natural gas, emitting more than 1500 million metric Tons of 𝐶𝑂! per year. Depending on regions and time of day, the cleanness of electricity significantly varies as more and more intermittent renewable energy resources, such as solar and wind, being added into the grid. With the growing awareness and regulations on GHG emissions, the need for accurate carbon measurement and technologies that reduces GHG for both the supply and demand side is ever-increasing. To optimally control demand-side users such as buildings, balance supply and demand, and incorporate energy storage technologies to reduce overall GHG emissions, the real-time emission factor and its predictions play critical roles. Here, we use the open-source real-time electricity GHG Emission Factor that covers all states in the US and four sample buildings’ demand data from Nantum OS across different regions to propose an optimization framework for potential emission reduction through load shifting. This study highlights the importance to raise awareness, monitor, and account for realtime GHG emissions. Furthermore, it proves the viability to control buildings with electric energy storage system to reduce carbon emissions for demand-side users.
Gulai Shen, Hongling Li, Gurpreet Singh, Amir Behjat, Gerald Toge, Souroush Senemmar, Cong Feng, Jie Zhang, Ali Mehmani
Research and Development, Nantum AI
The University of Texas at Dallas
National Renewable Energy Laboratory (NREL)
Electric power generation contributes to the second largest share of greenhouse gas (GHG) emissions in the US. The direct and indirect carbon emissions created from generating electricity vary from resources of generation, and power plant efficiency. Approximately 60% of the electricity comes from burning fossil fuels, mostly coal and natural gas, emitting more than 1500 million metric tons of 𝐶𝑂 per year. Depending on region and time of day, the cleanness of electricity significantly varies as more and more intermittent renewable energy resources, such as solar and wind, are added to the grid. With the growing awareness and regulations on GHG emissions, the need for accurate carbon measurement and technologies that reduce GHG for both the supply and demand side is ever-increasing. To optimally control demand-side users such as buildings, balance supply and demand, and incorporate energy storage technologies to reduce overall GHG emissions, the real-time emission factor and its predictions play critical roles. Here, we use the open-source real-time electricity GHG Emission Factor that covers all states in the US and four sample buildings’ demand data from Nantum across different regions to propose an optimization framework for potential emission reduction through load shifting. This study highlights the importance of raising awareness, monitoring, and accounting for real-time GHG emissions. Furthermore, it proves the viability of controlling buildings with electric energy storage systems to reduce carbon emissions for demand-side users.
Physics-Infused Retrofit Isolation Measurement and Verification for AI-Driven Energy Application
Tracking energy efficiency improvements, meeting environmental targets, and promoting sustainability practices are possible through accurate measurement and verification. In this paper, we offer a retrofit isolation measure and verification technology founded on the IPMVP standard of measuring the impact of different AI-driven ECMs, anomaly detection, and notification services. This technology uses physics-infused machine-learning models that are constructed using static and dynamic data. The key attributes of this scalable and open-source technology lie not only in its ability to provide accurate measurements and validations of the target ECMs, but also in its robustness to common non-routine adjustments such as changes in occupancy, alterations in facility size (e.g., added square footage), variations in space type or usage, increase in energy demand(e.g., new IT centers, additional plug loads, and new data centers), as well as adjustments in zone temperature set points.
Amir Behjat, Gulai Shen, Gurpreet Singh, Gerald Toge, Ali Mehmani
Research and Development, Nantum AI
Tracking energy efficiency improvements, meeting environmental targets, and promoting sustainability practices are possible through accurate measurement and verification. In this paper, we offer a retrofit isolation measure and verification technology founded on the IPMVP standard of measuring the impact of different AI-driven ECMs, anomaly detection, and notification services. This technology uses physics-infused machine-learning models that are constructed using static and dynamic data. The key attributes of this scalable and open-source technology lie not only in its ability to provide accurate measurements and validations of the target ECMs, but also in its robustness to common non-routine adjustments such as changes in occupancy, alterations in facility size (e.g., added square footage), variations in space type or usage, increase in energy demand(e.g., new IT centers, additional plug loads, and new data centers), as well as adjustments in zone temperature set points.
Data-Enabled Building Energy Savings (D-E BES)
Building sector energy consumption represents a significant fraction of the overall energy consumption in urban communities. While there has been increasing focus on the development of smart environments to support energy savings in urban commercial buildings, the development of smart environments for the residential sector has been less common. Instead, urban residential energy-saving efforts have focused on improving a building's physical infrastructure, introducing energy-saving appliances, and motivating residents to adopt energy conservation practices. This paper illustrates that creating an affinity between a building resident's thermal preferences and a building apartment's unregulated thermal environment represents an alternative means of generating an energy-efficient environment for multi-family, residential buildings. Two years of 15-min interval summer data, obtained from smart cyber-physical systems installed in 310 apartments across two New York City buildings, is used to develop data-enabled (D-E) models of resident thermal preference and unregulated apartment temperatures. Both of these models use a linear mixed-effects approach. The alignment of optimal resident-apartment pairs is then formulated as an integer-programming problem to explore the building energy saving (BES) potential associated with minimizing the difference between unregulated apartment temperature and the residents' thermal preference based on summer cooling loads. The work shows that the energy saving potential vary with a building's physical characteristics as well as the variation in residents' thermal preferences. The work further demonstrates that both the unregulated temperature and the resident's preferred temperature in a given apartment are not only affected by outside temperature and external relative humidity, but also strongly affected by the apartment's geographic orientation. For the specific buildings considered, up to 28% cooling energy saving is possible with full alignment. The paper provides all building monitoring data as an open source data set.
Deep Learning-Based Real-Time Building Occupancy Detection Using AMI Data
Building occupancy patterns facilitate successful development of the smart grid by enhancing building-to-grid integration efficiencies. Current occupancy detection is limited by the lack of widely deployed non-intrusive sensors and the insufficient learning power of shallow machine learning algorithms. This paper seeks to detect real-time building occupancy from Advanced Metering Infrastructure (AMI) data based on a deep learning architecture. The developed deep learning model consists of a convolutional neural network (CNN) and a long short-term memory (LSTM) network. Specifically, a CNN with convolutional and max-pooling layers extracts spatial features in the AMI data. Then, the forward and backward dependencies within the CNN feature maps are learned by a bidirectional LSTM (BiLSTM) structure with three hidden layers. Case studies based on a publicly available dataset show that the developed CNN-BiLSTM model consistently and robustly outperforms the state-of-the-art machine learning classifiers and other advanced deep learning architectures with around 90% occupancy detection accuracy and high detection confidence.
Indoor environmental wellness index (IEW-Index): Towards intelligent building systems automation and optimization
The impact of indoor environment quality (IEQ) on occupants’ wellness and productivity is substantial. Simultaneously, due to buildings’ significant role as one of the largest energy consumers, there is a pressing need for improved energy efficiency and intelligence in building design and operation. This paper introduces a novel indoor environmental wellness index (IEW-Index) as a real-time key performance indicator (KPI) derived from controllable factors associated with wellness. The main objective of this index is to develop a universally applicable metric for IEQ, incorporate real-time data and personalized settings, and facilitate the real-time optimization of building control systems. In addition, a novel framework based on a multi-agent system (MAS) for building control systems is introduced, encompassing a detailed design of components and communication protocols. The IEW-Index’s effectiveness has been successfully demonstrated through three real-world case studies formulated using the developed MAS framework. The findings indicate a direction for creating a sustainable building automation system that is intelligent and autonomous.
Concurrent optimization of thermal and electric storage in commercial buildings to reduce operating cost and demand peaks under time-of-use tariffs
Demand-side management (DSM) in response to market-based electricity tariffs can potentially increase the efficiency and reliability of the electric power grid. This study introduces a novel, one-day-ahead DSM framework which optimizes temperature setpoints and battery dispatch in office buildings, subject to a time-varying and/or demand-based electricity tariff. To reflect real world implementation, our framework operates in two-steps. First, during the passive, battery-only DSM optimization, historical weather and electricity load data for a given building are used to determine its optimal battery capacity. Second, once the battery has been installed, a one-day-ahead, real-time DSM algorithm optimizes both the building’s daily temperature setpoints and the battery's charge/discharge pattern. The optimization objective is to minimize the total operating cost (tariff charges and battery system) while still satisfying occupants’ thermal comfort. Using a case study with a medium-fidelity electric load model for a standard office building, the performance of the proposed framework is validated by quantifying savings in operating cost, reduction of monthly grid peak loads, and the achieved human occupant comfort. To illustrate the advantage of optimizing temperature setpoints and battery dispatch concurrently, the combined performance is compared with that achieved by standalone DSM (i.e., using only battery dispatch or only temperature setpoints). We found that concurrent optimization can reduce a building's monthly peak demand on the grid by up to 26%. Electricity tariff charges are reduced by 11%, more than is required to offset storage costs, thus providing an overall profit to building operators who use such DSM. Payback time is approximately 5 years.
Residential electricity conservation in response to auto-generated, multi-featured, personalized eco-feedback designed for large-scale applications with utilities
While past research has shown that providing residents with feedback about their electricity usage can reduce demand and its associated environmental burdens, some questions remain regarding what makes such feedback most effective. We followed the electricity usage of 36 residents who each received 14 feedback messages over 2 months. Using approaches borrowed from Natural-Language-Processing, feedbacks were generated automatically, using 10 features in random combinations. Unlike in previous studies, each resident received varying types of messages over time. In 504 observations, the average prompted reduction in electricity usage was 11 ± 3%, compared to a control group of 89 residents who received no messages. Feedback types prompting the largest reductions were self-comparisons with one’s own earlier usage (average reduction 14%) and messages of high variety from one feedback-cycle to the next (average reduction 16%). Comparisons with neighbors did not prompt higher reductions on average. Instead, they prompted reductions only when a resident’s recent usage happened to be higher than the average usage of neighbors, and increases when the reverse was true. This behavior was exhibited by all residents and is likely explained by a norm-conforming mean reversion of residents to their neighbors’ average usage, rather than an anti-conform “boomerang” behavior previously suggested in similar contexts.
MFRED, 10 second interval real and reactive power for groups of 390 US apartments of varying size and vintage
Building electricity is a major component of global energy use and its environmental impacts. Detailed data on residential electricity use have many interrelated research applications, from energy conservation to non-intrusive load monitoring, energy storage, integration of renewables, and electric vs. fossil-based heating. The dataset presented here, Multifamily Residential Electricity Dataset (MFRED), contains the electricity use of 390 apartments, ranging from studios to four-bedroom units. All apartments are located in the Northeastern United States (IECC-climate-zone 4 A), but differ in their heating/cooling system and construction year (early to late 20th century). To adhere to privacy guidelines, data were averaged across 15 apartments each, based on annual electricity use. MFRED includes real and reactive power, at 10-second resolution, for January to December 2019 (246 million data points). The annual average real power per apartment is 343 W (3.27 W/m2 of floor area), with strong variation between seasons and apartment size. Considering its large number of apartments, high time resolution, real and reactive power, and 12-month duration, MFRED is currently unique for the multifamily-sector.
Adaptive in situ model refinement for surrogate-augmented population-based optimization
In surrogate-based optimization (SBO), the deception issues associated with the low fidelity of the surrogate model can be dealt with in situ model refinement that uses infill points during optimization. However, there is a lack of model refinement methods that are both independent of the choice of surrogate model (neural networks, radial basis functions, Kriging, etc.) and provides a methodical approach to preserve the fidelity of the search dynamics, especially in the case of population-based heuristic optimization processes. This paper presents an adaptive model refinement (AMR) approach to fill this important gap. Therein, the question of when to refine the surrogate model is answered by a novel hypothesis testing concept that compares the distribution of model error and distribution of function improvement over iterations. These distributions are respectively computed via a probabilistic cross-validation approach and by leveraging the probabilistic improvement information uniquely afforded by population-based algorithms such as particle swarm optimization. Moreover, the AMR method identifies the size of the batch of infill points needed for refinement. Numerical experiments performed on multiple benchmark functions and an optimal (building energy) planning problem demonstrate AMR’s ability to preserve computational efficiency of the SBO process while providing solutions of more attractive fidelity than those provisioned by a standard SBO approach.
Concurrent surrogate model selection (COSMOS): optimizing model type, kernel function, and hyper-parameters
This paper presents an automated surrogate model selection framework called the Concurrent Surrogate Model Selection or COSMOS. Unlike most existing techniques, COSMOS coherently operates at three levels, namely: 1) selecting the model type (e.g., RBF or Kriging), 2) selecting the kernel function type (e.g., cubic or multiquadric kernel in RBF), and 3) determining the optimal values of the typically user-prescribed hyper-parameters (e.g., shape parameter in RBF). The quality of the models is determined and compared using measures of median and maximum error, given by the Predictive Estimation of Model Fidelity (PEMF) method. PEMF is a robust implementation of sequential k-fold cross-validation. The selection process undertakes either a cascaded approach over the three levels or a more computationally-efficient one-step approach that solves a mixed-integer nonlinear programming problem. Genetic algorithms are used to perform the optimal selection. Application of COSMOS to benchmark test functions resulted in optimal model choices that agree well with those given by analyzing the model errors on a large set of additional test points. For the four analytical benchmark problems and three practical engineering applications – airfoil design, window heat transfer modeling, and building energy modeling – diverse forms of models/kernels are observed to be selected as optimal choices. These observations further establish the need for automated multi-level model selection that is also guided by dependable measures of model fidelity.