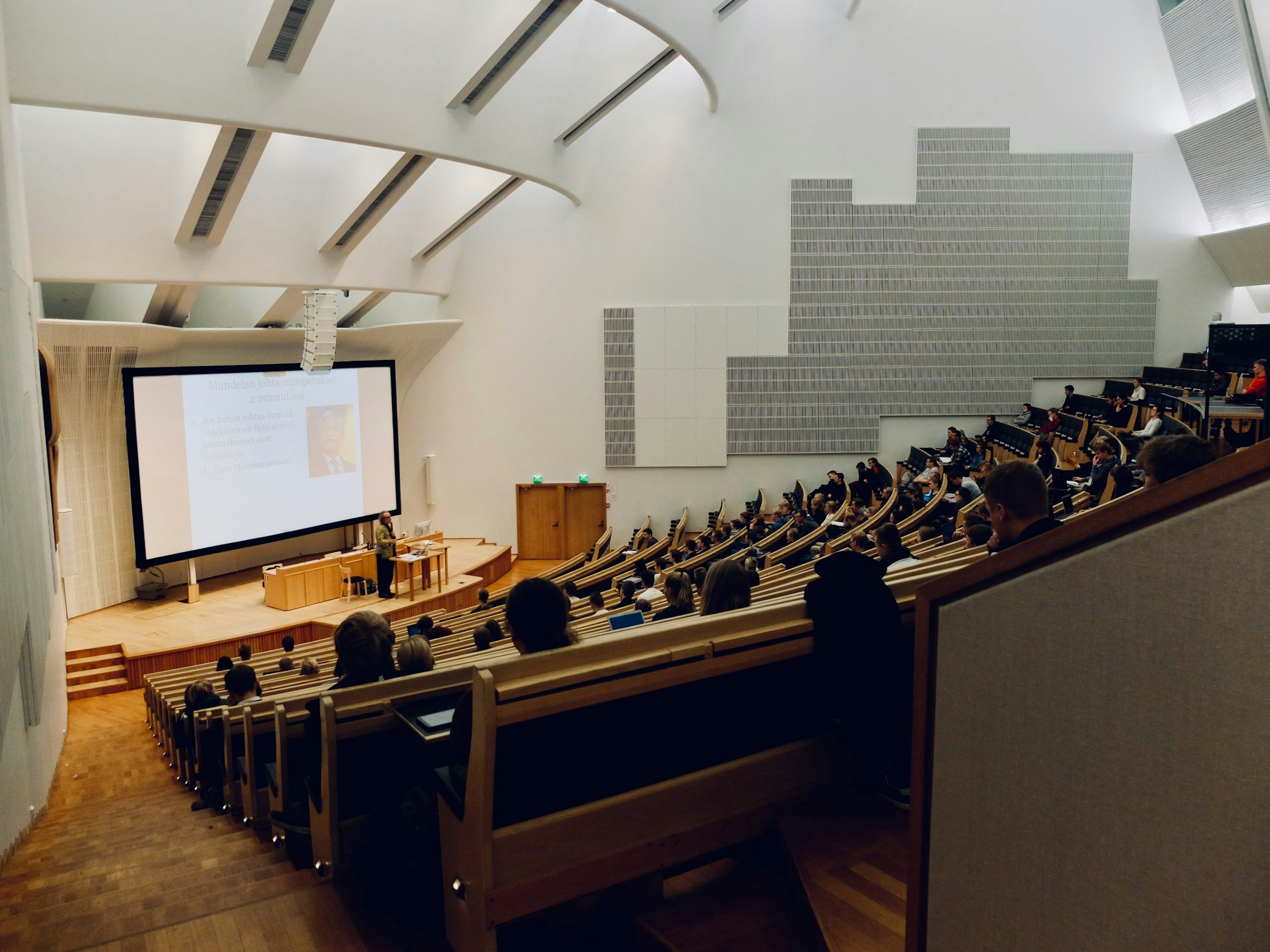
Academic Research
Our team are experts at building science, machine learning, and artificial intelligence. Our Research & Development team shares our research in academic journals.
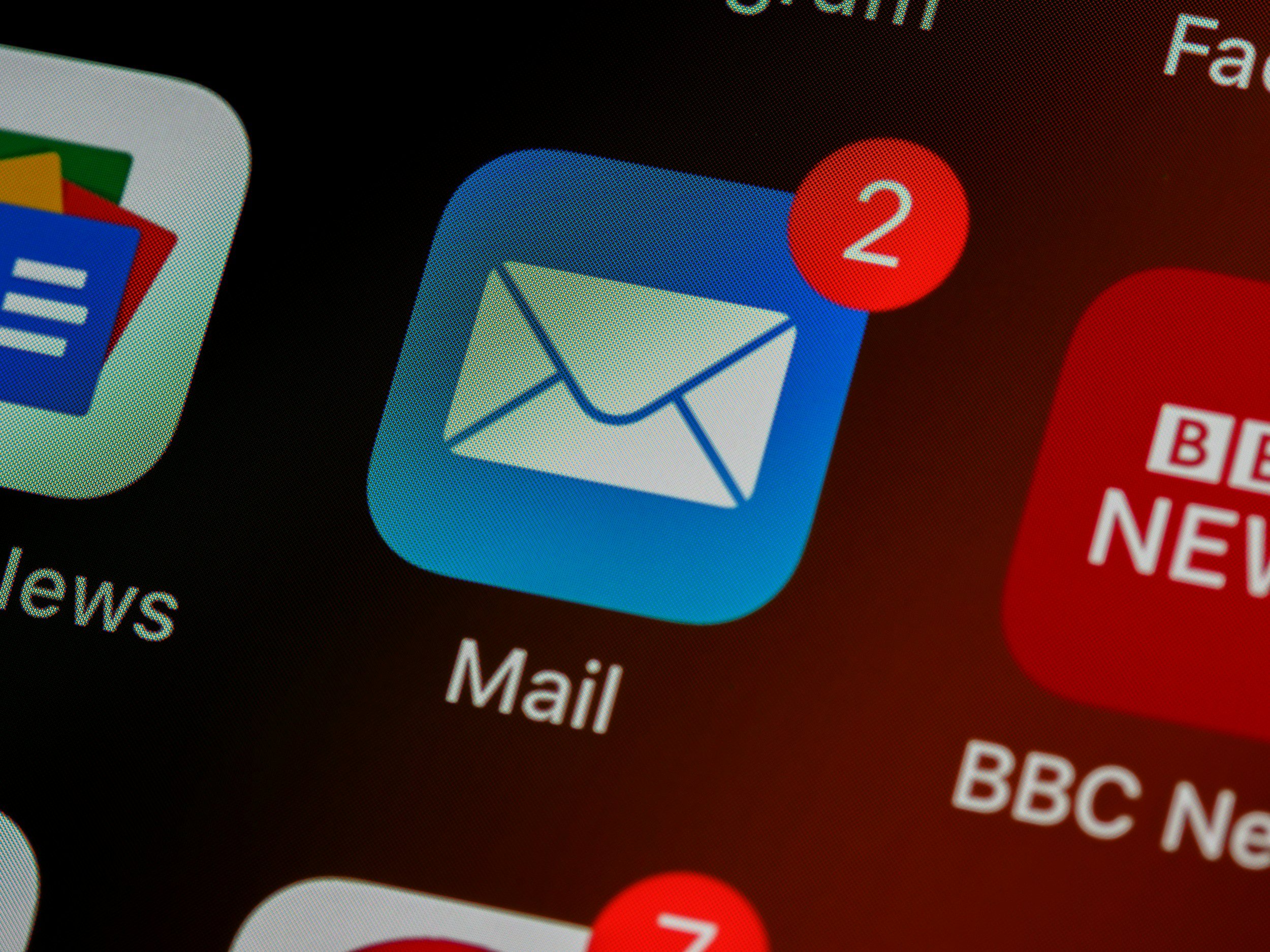
Newsletter Subscription
Sign up to stay informed with the latest updates, exclusive news, future events & opportunities, and valuable insights delivered directly to your inbox.
Deep Learning-Based Real-Time Building Occupancy Detection Using AMI Data
Building occupancy patterns facilitate successful development of the smart grid by enhancing building-to-grid integration efficiencies. Current occupancy detection is limited by the lack of widely deployed non-intrusive sensors and the insufficient learning power of shallow machine learning algorithms. This paper seeks to detect real-time building occupancy from Advanced Metering Infrastructure (AMI) data based on a deep learning architecture. The developed deep learning model consists of a convolutional neural network (CNN) and a long short-term memory (LSTM) network. Specifically, a CNN with convolutional and max-pooling layers extracts spatial features in the AMI data. Then, the forward and backward dependencies within the CNN feature maps are learned by a bidirectional LSTM (BiLSTM) structure with three hidden layers. Case studies based on a publicly available dataset show that the developed CNN-BiLSTM model consistently and robustly outperforms the state-of-the-art machine learning classifiers and other advanced deep learning architectures with around 90% occupancy detection accuracy and high detection confidence.