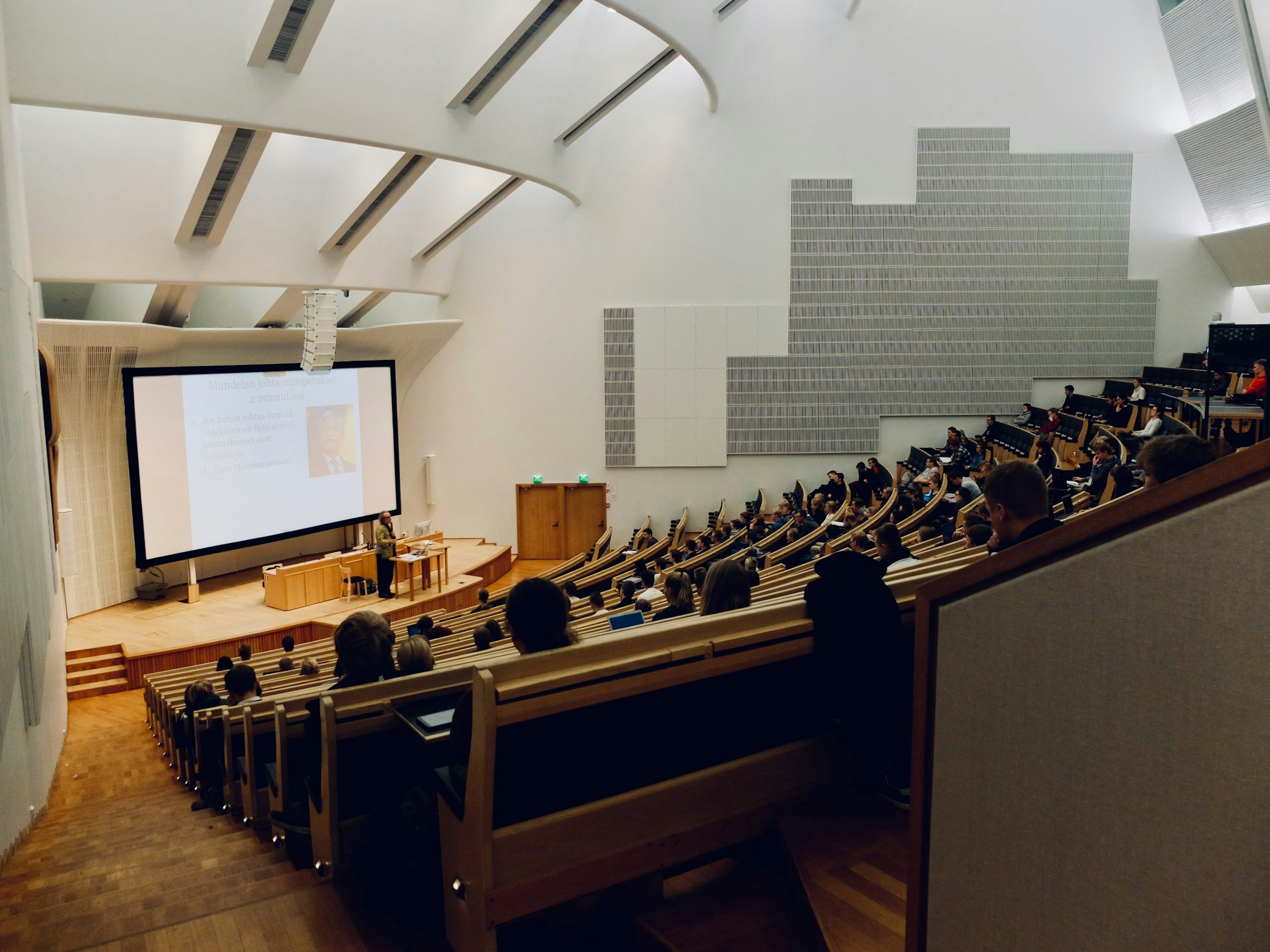
Academic Research
Our team are experts at building science, machine learning, and artificial intelligence. Our Research & Development team shares our research in academic journals.
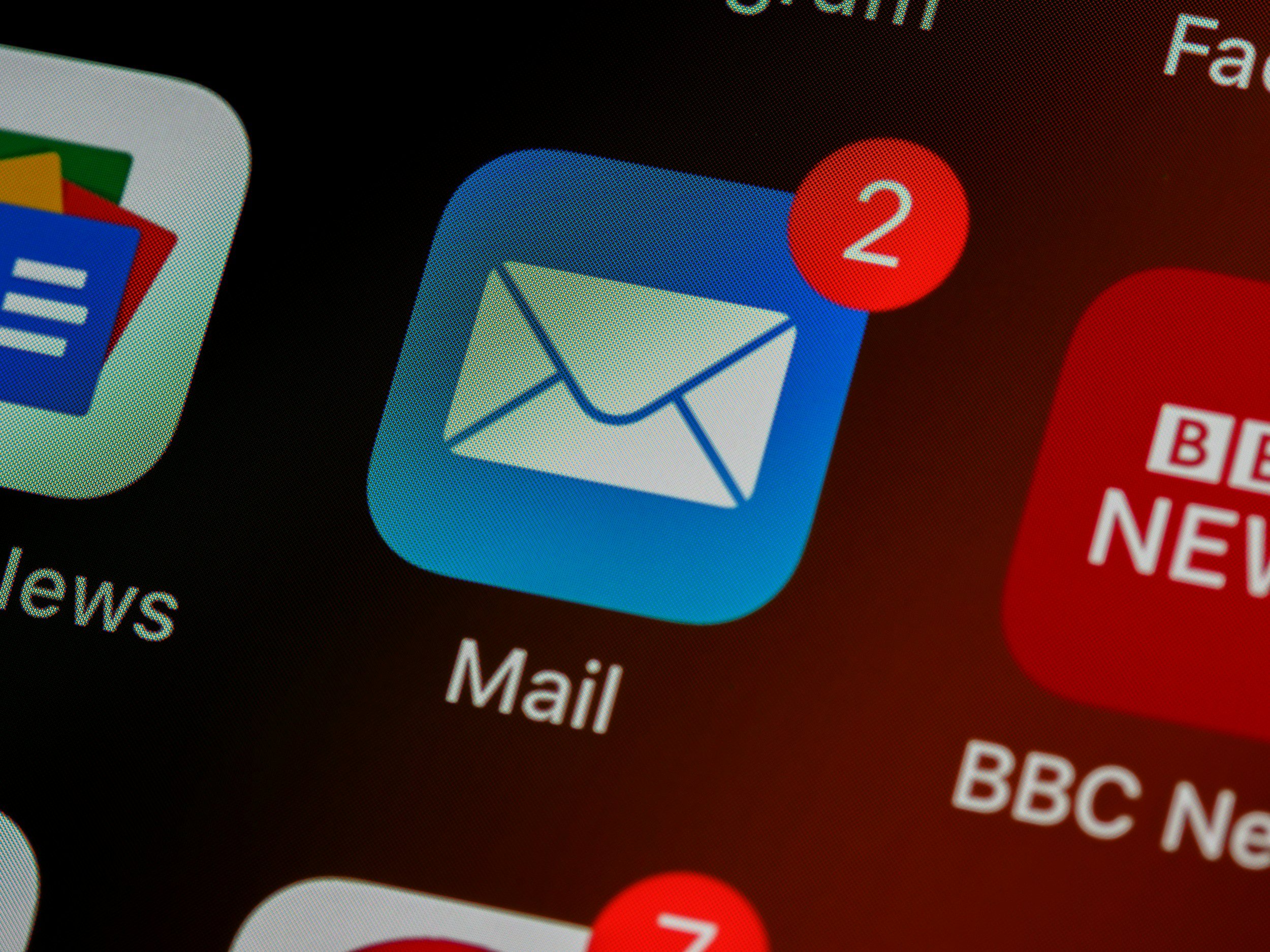
Newsletter Subscription
Sign up to stay informed with the latest updates, exclusive news, future events & opportunities, and valuable insights delivered directly to your inbox.
Demand-Side Management Under Real-Time Greenhouse Gas Emission Factor for Electricity
Electric power generation contributes to the second largest share of greenhouse gas (GHG) emissions in the US. The direct and indirect carbon emissions created from generating electricity vary from resources of generation, and power plant efficiency. Approximately 60% of the electricity comes from burning fossil fuels, mostly coal and natural gas, emitting more than 1500 million metric Tons of 𝐶𝑂! per year. Depending on regions and time of day, the cleanness of electricity significantly varies as more and more intermittent renewable energy resources, such as solar and wind, being added into the grid. With the growing awareness and regulations on GHG emissions, the need for accurate carbon measurement and technologies that reduces GHG for both the supply and demand side is ever-increasing. To optimally control demand-side users such as buildings, balance supply and demand, and incorporate energy storage technologies to reduce overall GHG emissions, the real-time emission factor and its predictions play critical roles. Here, we use the open-source real-time electricity GHG Emission Factor that covers all states in the US and four sample buildings’ demand data from Nantum OS across different regions to propose an optimization framework for potential emission reduction through load shifting. This study highlights the importance to raise awareness, monitor, and account for realtime GHG emissions. Furthermore, it proves the viability to control buildings with electric energy storage system to reduce carbon emissions for demand-side users.
Gulai Shen, Hongling Li, Gurpreet Singh, Amir Behjat, Gerald Toge, Souroush Senemmar, Cong Feng, Jie Zhang, Ali Mehmani
Research and Development, Nantum AI
The University of Texas at Dallas
National Renewable Energy Laboratory (NREL)
Electric power generation contributes to the second largest share of greenhouse gas (GHG) emissions in the US. The direct and indirect carbon emissions created from generating electricity vary from resources of generation, and power plant efficiency. Approximately 60% of the electricity comes from burning fossil fuels, mostly coal and natural gas, emitting more than 1500 million metric tons of 𝐶𝑂 per year. Depending on region and time of day, the cleanness of electricity significantly varies as more and more intermittent renewable energy resources, such as solar and wind, are added to the grid. With the growing awareness and regulations on GHG emissions, the need for accurate carbon measurement and technologies that reduce GHG for both the supply and demand side is ever-increasing. To optimally control demand-side users such as buildings, balance supply and demand, and incorporate energy storage technologies to reduce overall GHG emissions, the real-time emission factor and its predictions play critical roles. Here, we use the open-source real-time electricity GHG Emission Factor that covers all states in the US and four sample buildings’ demand data from Nantum across different regions to propose an optimization framework for potential emission reduction through load shifting. This study highlights the importance of raising awareness, monitoring, and accounting for real-time GHG emissions. Furthermore, it proves the viability of controlling buildings with electric energy storage systems to reduce carbon emissions for demand-side users.
Physics-Infused Retrofit Isolation Measurement and Verification for AI-Driven Energy Application
Tracking energy efficiency improvements, meeting environmental targets, and promoting sustainability practices are possible through accurate measurement and verification. In this paper, we offer a retrofit isolation measure and verification technology founded on the IPMVP standard of measuring the impact of different AI-driven ECMs, anomaly detection, and notification services. This technology uses physics-infused machine-learning models that are constructed using static and dynamic data. The key attributes of this scalable and open-source technology lie not only in its ability to provide accurate measurements and validations of the target ECMs, but also in its robustness to common non-routine adjustments such as changes in occupancy, alterations in facility size (e.g., added square footage), variations in space type or usage, increase in energy demand(e.g., new IT centers, additional plug loads, and new data centers), as well as adjustments in zone temperature set points.
Amir Behjat, Gulai Shen, Gurpreet Singh, Gerald Toge, Ali Mehmani
Research and Development, Nantum AI
Tracking energy efficiency improvements, meeting environmental targets, and promoting sustainability practices are possible through accurate measurement and verification. In this paper, we offer a retrofit isolation measure and verification technology founded on the IPMVP standard of measuring the impact of different AI-driven ECMs, anomaly detection, and notification services. This technology uses physics-infused machine-learning models that are constructed using static and dynamic data. The key attributes of this scalable and open-source technology lie not only in its ability to provide accurate measurements and validations of the target ECMs, but also in its robustness to common non-routine adjustments such as changes in occupancy, alterations in facility size (e.g., added square footage), variations in space type or usage, increase in energy demand(e.g., new IT centers, additional plug loads, and new data centers), as well as adjustments in zone temperature set points.