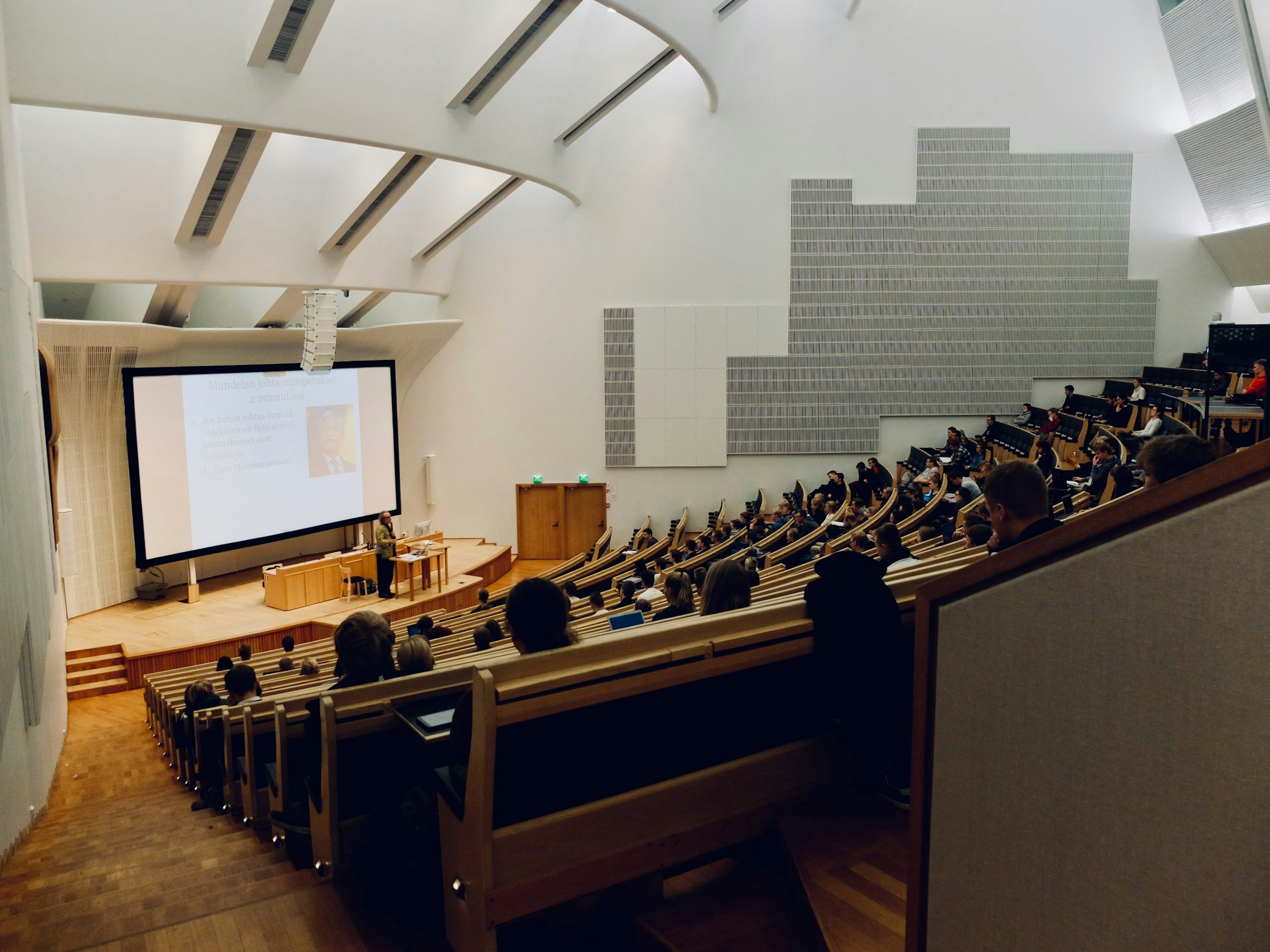
Academic Research
Our team are experts at building science, machine learning, and artificial intelligence. Our Research & Development team shares our research in academic journals.
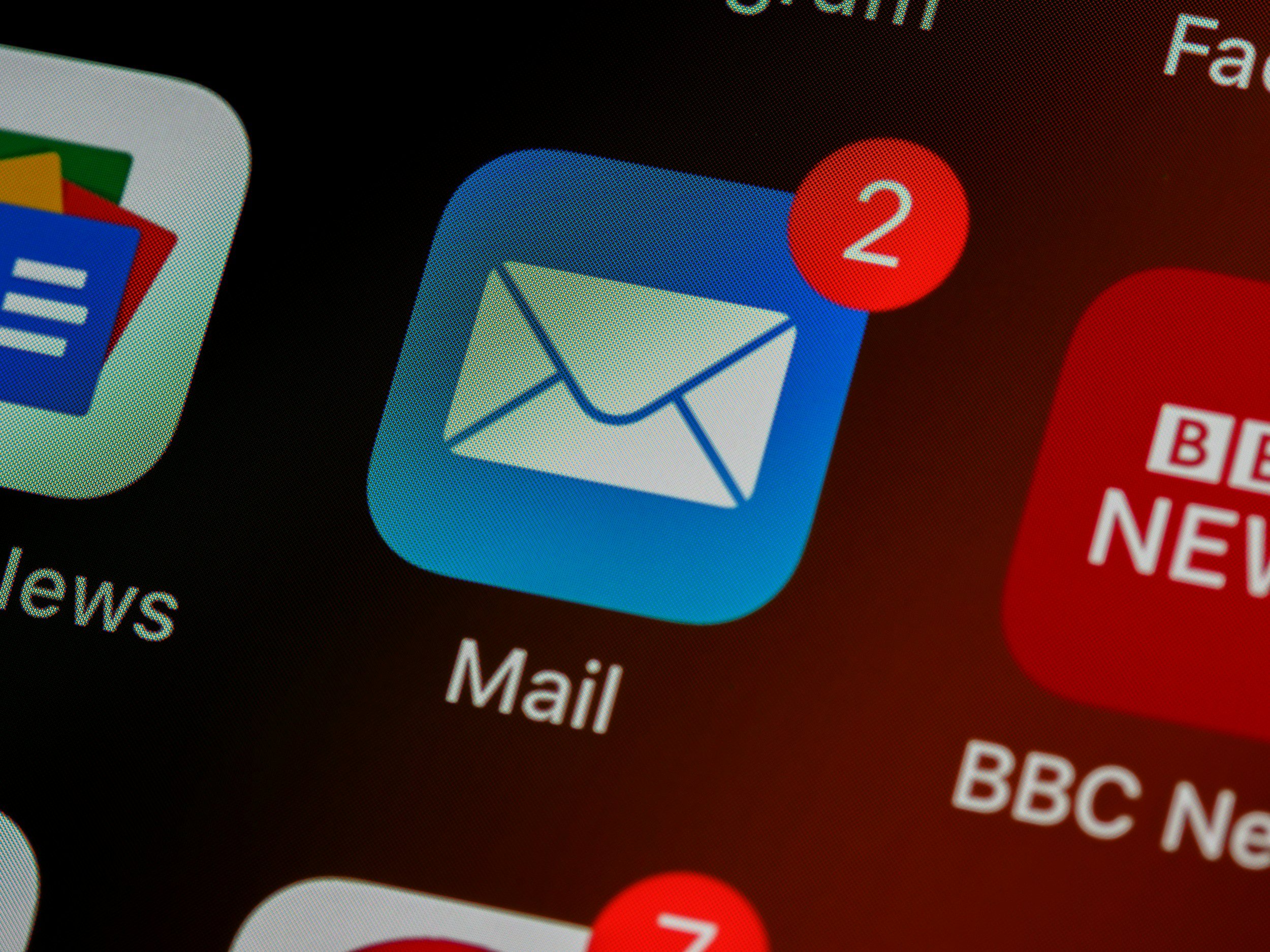
Newsletter Subscription
Sign up to stay informed with the latest updates, exclusive news, future events & opportunities, and valuable insights delivered directly to your inbox.
Real-time Greenhouse Gas Emission Intensity Informed Demand-side Load Regulation For Power Grid Decarbonization
The US targets net-zero-carbon electricity by 2035, emphasizing the need for both supply- and demand-side strategies in the power sector. Our study focuses on demand-side carbon reduction via load regulation, using real-time greenhouse gas emission data to optimize electricity use without reducing overall consumption. Our multi-scenario analysis indicates significant potential for carbon footprint reduction by adapting strategies to specific grid characteristics. For example, California could have 32.58% more emission reduction with annual instead of quarterly optimization. In grids with diverse generation resources, expanding adjustment ranges from ±5% to ±8% can boost carbon reductions from 1.19% to 1.64%. Our results also reveal low greenhouse gas intensity fluctuation areas as more cost sensitive in electricity consumption than high greenhouse gas intensity fluctuation areas, providing essential insights for policymakers.
Honglin Li, Gulai Shen, Souroush Senemmar, Ali Mehmani, Jie Zhang
Nantum AI, New York, NY, United States of America
Harvard University, Cambridge, MA, United States of America
The University of Texas at Dallas, Dallas, TX, United States of America
Science for Society
The United States aims to achieve net-zero carbon electricity by 2035, necessitating innovative approaches to both energy consumption and generation. Our research examines the use of real-time greenhouse gas (GHG) emissions data to adjust electricity usage, thereby reducing the carbon footprint without decreasing overall energy consumption. This strategy proves to be particularly effective in regions rich in renewable energy, where annual optimization could lead to emission reductions of over 30%. Scaling this strategy presents both opportunities and challenges. Specifically, different power grids will require tailored approaches; for example, grids with a mix of energy sources could widen consumption adjustment ranges to enhance carbon reduction. Conversely, areas with varying GHG intensity fluctuations may respond differently to the costs of load regulation, necessitating customized policies. This approach provides a scalable and actionable solution for US decarbonization efforts, leveraging real-time data to achieve significant environmental impact.
Highlights
A demand-side load regulation model using real-time greenhouse gas intensity is proposed.
Three different dimensions of demand-side regulation strategies are proposed.
Demand-side regulation strategies for different energy system types are analyzed.
Summary
The US targets net-zero-carbon electricity by 2035, emphasizing the need for both supply- and demand-side strategies in the power sector. Our study focuses on demand-side carbon reduction via load regulation, using real-time greenhouse gas emission data to optimize electricity use without reducing overall consumption. Our multi-scenario analysis indicates significant potential for carbon footprint reduction by adapting strategies to specific grid characteristics. For example, California could have 32.58% more emission reduction with annual instead of quarterly optimization. In grids with diverse generation resources, expanding adjustment ranges from ±5% to ±8% can boost carbon reductions from 1.19% to 1.64%. Our results also reveal low greenhouse gas intensity fluctuation areas as more cost sensitive in electricity consumption than high greenhouse gas intensity fluctuation areas, providing essential insights for policymakers.